Revolutionizing Data Annotation with Video Labeling Tool Machine Learning
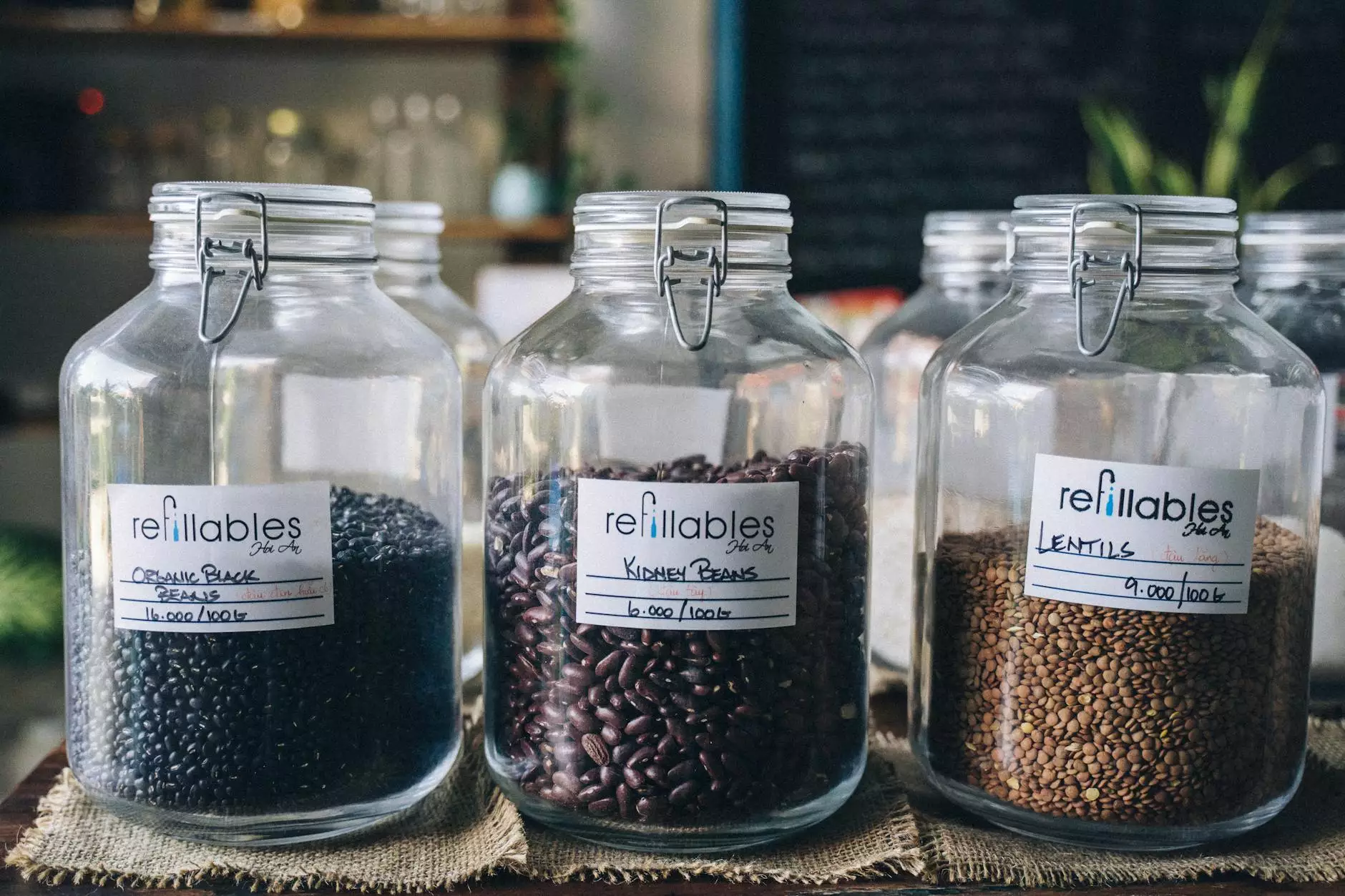
In the fast-paced world of data annotation, the use of a video labeling tool powered by machine learning is transforming the landscape of how businesses operate. With the increasing requirement for large amounts of labeled data for training AI models, efficient and scalable solutions are becoming paramount. This article delves into the various aspects of video labeling tools, their importance in the annotation process, and how they integrate with machine learning to optimize business operations.
Understanding Video Labeling Tools
Video labeling tools are specialized software designed to annotate videos for various applications, including autonomous driving, surveillance, and content moderation. These tools allow users to label objects, actions, and events within video frames, providing detailed data that is essential for training machine learning models. The core functionality of a video labeling tool includes:
- Frame-by-frame analysis: Enables users to examine and label each frame in a video.
- Object tracking: Facilitates the continuous tracking of objects across multiple frames.
- Action recognition: Identifies and labels specific actions or behaviors within a video.
- Integration with machine learning: Implements algorithms that improve the speed and accuracy of the labeling process.
The Role of Machine Learning in Video Labeling
Machine learning has a pivotal role in enhancing the capabilities of video labeling tools. By leveraging advanced algorithms, machine learning models can automate significant portions of the annotation process, resulting in increased efficiency and accuracy. Key benefits of integrating machine learning into video labeling include:
1. Increased Efficiency
Manual video labeling is a time-consuming task, especially for large datasets. Machine learning algorithms can automate mundane tasks, allowing human annotators to focus on more complex and nuanced labeling requirements. This automation speeds up the data preparation phase, leading to faster deployment of AI models.
2. Higher Accuracy
With the continuous training of machine learning models, the accuracy of predictions and labels improves over time. These models learn from previous annotations and make more informed predictions, reducing human error in the labeling process.
3. Scalability
As businesses grow, so do their data requirements. Machine learning-driven video labeling tools can easily scale to accommodate increasing data volumes without a proportional increase in manual labor.
Key Features of Advanced Video Labeling Tools
To truly grasp the capabilities of modern video labeling tools, it’s essential to understand their key features. Here are some of the advanced functionalities offered by these cutting-edge tools:
1. User-Friendly Interface
A user-friendly interface is crucial for any annotation platform. Video labeling tools typically offer intuitive designs that enable users to navigate effortlessly, making the labeling process straightforward, even for those with limited technical experience.
2. Collaborative Annotation
Many video labeling tools support collaborative work, allowing teams to annotate videos simultaneously. This feature is particularly beneficial for large projects where multiple annotators can work together in real-time, improving project turnaround times.
3. Customizable Annotation Schemes
Businesses have unique data needs, which means that customizable annotation schemes are essential. Advanced tools allow users to create specific labels and categories tailored to their requirements, providing flexibility and precision in data annotation.
4. Integration with Other Tools
Seamless integration with other data processing tools and data management platforms is a significant advantage. This interoperability enables efficient workflows and reduces the need for duplicate data entry across systems.
Applications of Video Labeling Tools in Various Industries
The applications of video labeling tools are vast and span across numerous industries. Here are some notable areas where these tools are making a significant impact:
1. Autonomous Vehicles
In the field of autonomous driving, video labeling tools are used to annotate driving scenarios, object detection, and traffic sign recognition. These labeled datasets are critical for training self-driving algorithms, ensuring safety and efficiency in autonomous transportation.
2. Security and Surveillance
Video surveillance systems utilize labeling tools to identify suspicious activities, track individuals, and provide detailed reports of incidents. By automating the analysis of surveillance footage, businesses can enhance security measures and operational efficiency.
3. Content Moderation
In content moderation for online platforms, video labeling ensures compliance with regulations and community standards. By accurately tagging inappropriate content, companies can maintain safe environments for their users.
4. Healthcare
In healthcare, video labeling tools assist in the analysis of medical videos, such as surgeries or patient monitoring. By annotating important events or behaviors, healthcare providers can improve training, diagnostics, and patient outcomes.
The Future of Video Labeling Tools with Machine Learning
The future of video labeling tools is bright, especially as advancements in machine learning continue to evolve. As artificial intelligence technologies improve, we can anticipate the following trends in the coming years:
1. Enhanced Automation
Future video labeling tools will increasingly leverage machine learning for automation, reducing the need for human intervention. As algorithms become more sophisticated, they will be able to handle complex labeling tasks with minimal guidance.
2. Real-Time Processing
Real-time video analysis will become a standard feature, enabling instant labeling and feedback. This capability will be particularly valuable in fast-paced environments like surveillance and live streaming.
3. Improved User Interaction
We can expect to see advancements in how users interact with video labeling tools, including augmented reality (AR) and virtual reality (VR) interfaces that provide immersive experiences for annotators.
4. Greater Customization and Flexibility
Future tools will offer even more customization options, allowing businesses to adapt the tools to their specific workflow needs, promoting efficiency and effectiveness.
Conclusion
In conclusion, adopting a video labeling tool machine learning solution is essential for any business looking to harness the power of AI and machine learning. Not only do these tools streamline the data annotation process, they also provide enhanced accuracy and scalability to meet growing data needs. As seen across various industries, the integration of advanced video labeling tools is a key factor in driving innovation and maintaining competitive advantage. For businesses looking to enhance their data annotation efforts, exploring the offerings at Keylabs.ai is a vital next step towards achieving operational excellence.
Take the Next Step in Data Annotation
If you're ready to elevate your data annotation process with a robust video labeling tool backed by advanced machine learning, Keylabs.ai is here to help. Our platform offers state-of-the-art solutions designed to meet the needs of any business seeking to lead in their respective fields.