Understanding the Importance of Bounding Boxes in Data Annotation
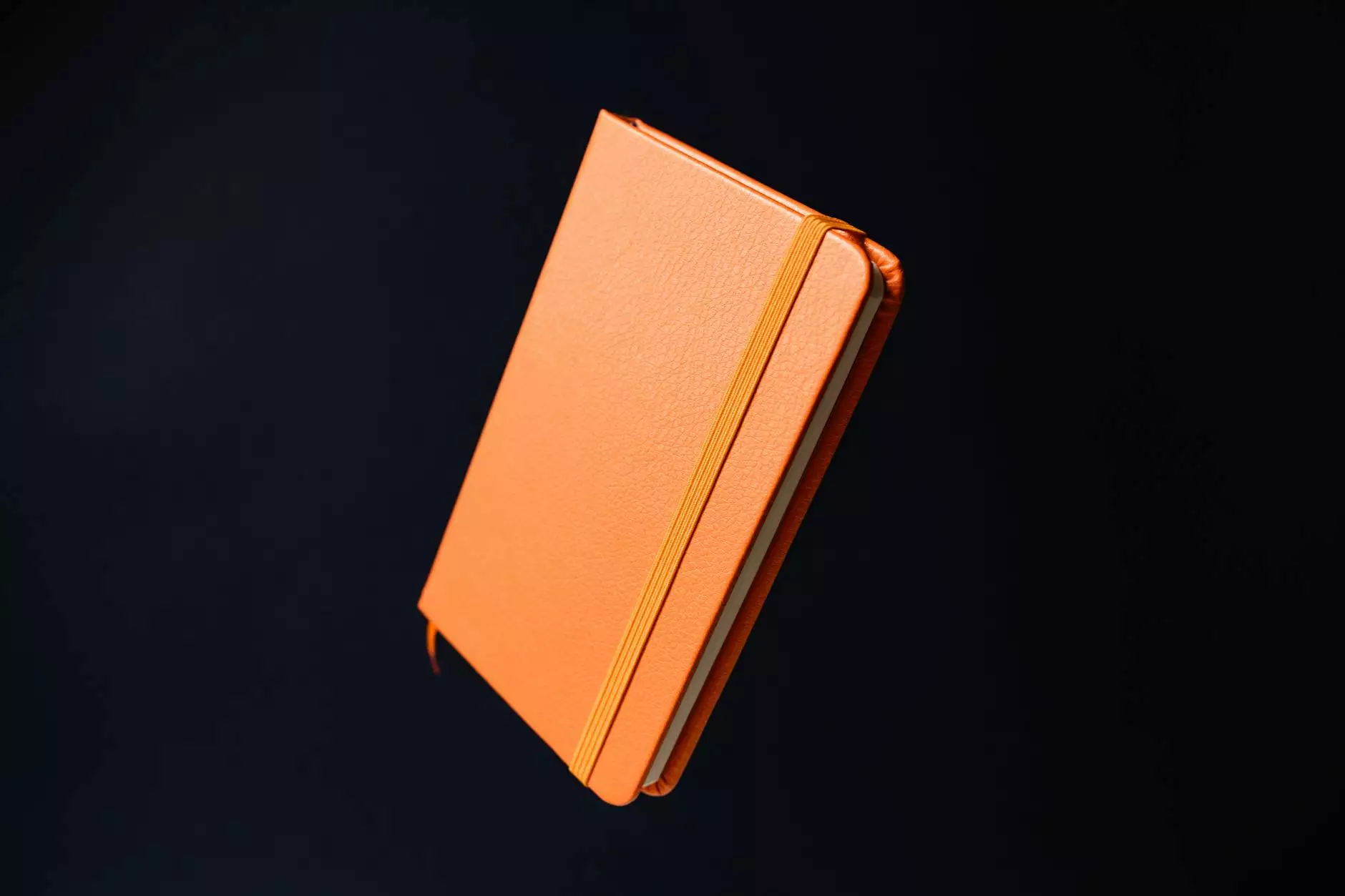
In today's data-driven world, businesses are leveraging artificial intelligence and machine learning technologies to enhance their operational efficiency. A significant part of this transformation involves the use of data annotation tools and platforms, where one critical element often comes into play: bounding boxes. This article delves into the intricacies of bounding boxes, their applications, importance, and how they can be effectively utilized within the context of data annotation.
What are Bounding Boxes?
Bounding boxes are rectangular boxes drawn around objects of interest in digital images. They serve as either a label or a support tool for machine learning algorithms, enabling models to recognize, track, and understand visual data. The utility of bounding boxes extends to various fields, including computer vision, autonomous driving, drones, and medical imaging.
The Role of Bounding Boxes in Data Annotation
Data annotation plays a crucial role in enabling machine learning models to learn effectively. By marking and categorizing data for training, data scientists and engineers can ensure that their algorithms are exposed to high-quality information. Within this process, bounding boxes fulfill specific functions:
- Object Localization: They help in pinpointing the exact location of objects in a scene.
- Class Labeling: Each bounding box can be assigned a label, informing the model what the object is (e.g., "car", "person").
- Model Training: Provides a rich dataset for teaching models to recognize patterns and features associated with specific objects.
Applications of Bounding Boxes
Bounding boxes are widely utilized across various industries. Below are some key applications:
1. Autonomous Vehicles
In autonomous driving technology, bounding boxes are essential for identifying pedestrians, vehicles, traffic lights, and other critical elements on the road. By accurately locating these objects, the technology can make informed decisions that enhance safety and efficiency.
2. Retail and Inventory Management
In the retail sector, stores use computer vision systems to manage inventory. Bounding boxes help track products on shelves, enabling real-time inventory checks and automated restocking alerts.
3. Facial Recognition Systems
Facial recognition technologies employ bounding boxes to locate and identify individual faces in images or video streams. This has widespread implications in security, social media, and personalized marketing.
4. Healthcare and Medical Imaging
In healthcare, bounding boxes are applied in medical imaging analysis for object segmentation (like tumors in scans), increasing diagnostic accuracy and aiding radiologists in decision-making processes.
Benefits of Using Bounding Boxes in Data Annotation
Implementing bounding boxes in your data annotation strategy offers numerous advantages:
- Enhanced Data Quality: Provides clear demarcation of objects, enhancing the quality of labeled data.
- Increased Model Accuracy: By properly labeling and identifying objects, models can achieve higher accuracy rates in tasks such as image classification and object detection.
- Efficiency in Data Preparation: Facilitates faster data preparation processes through automation and improved annotation workflows.
- Scalability: As datasets grow, bounding boxes allow for consistent and scalable labeling approaches across large volumes of images.
Best Practices for Implementing Bounding Boxes
To maximize the effectiveness of bounding boxes in your annotation projects, consider the following best practices:
1. Ensure Accurate Placement
When creating bounding boxes, ensure they tightly enclose the object in question without excessive whitespace. Accuracy in placement is crucial for model training.
2. Use Consistent Labeling Guidelines
Establish clear guidelines for labeling objects with bounding boxes to maintain consistency. This helps teams avoid confusion, ensuring that models receive uniform data.
3. Leverage Advanced Tools and Software
Utilize sophisticated data annotation tools that provide features for drawing and managing bounding boxes. Platforms like Keylabs.ai offer intuitive interfaces that enhance productivity and ensure quality.
4. Regularly Review and Update Annotations
Conduct periodic reviews of your data annotations to adjust for any discrepancies or changes in the field. Maintain flexibility to ensure your models are trained on the most relevant and accurate data.
The Future of Bounding Boxes in Data Annotation
The future of bounding boxes in data annotation looks promising as technology advances. With the rise of deep learning techniques and the integration of more sophisticated algorithms, we can expect the following trends:
- Integration with AI: Automatic generation and refinement of bounding boxes through AI algorithms will reduce manual annotation efforts.
- 3D Annotation: As technology progresses, we may see bounding boxes adapting to three-dimensional environments, enhancing object recognition capabilities in a more immersive manner.
- Real-Time Processing: Real-time annotation capabilities in dynamic environments such as video feeds will increase the applicability of bounding boxes in industries like surveillance and autonomous systems.
Conclusion
In a landscape powered by data, the role of bounding boxes in data annotation cannot be overstated. They are fundamental in training artificial intelligence systems, enabling accurate object detection and classification across a multitude of applications. As businesses continue to recognize the value of high-quality annotated data, implementing effective bounding box strategies will be essential for staying competitive and innovative in the realm of AI.
For businesses looking to enhance their data annotation efforts, platforms like Keylabs.ai provide cutting-edge tools that streamline the creation and management of bounding boxes. By investing in proper data annotation practices today, organizations can pave the way for significantly improved outcomes in their artificial intelligence and machine learning initiatives.