Unlocking the Power of Labeling Tools for Machine Learning
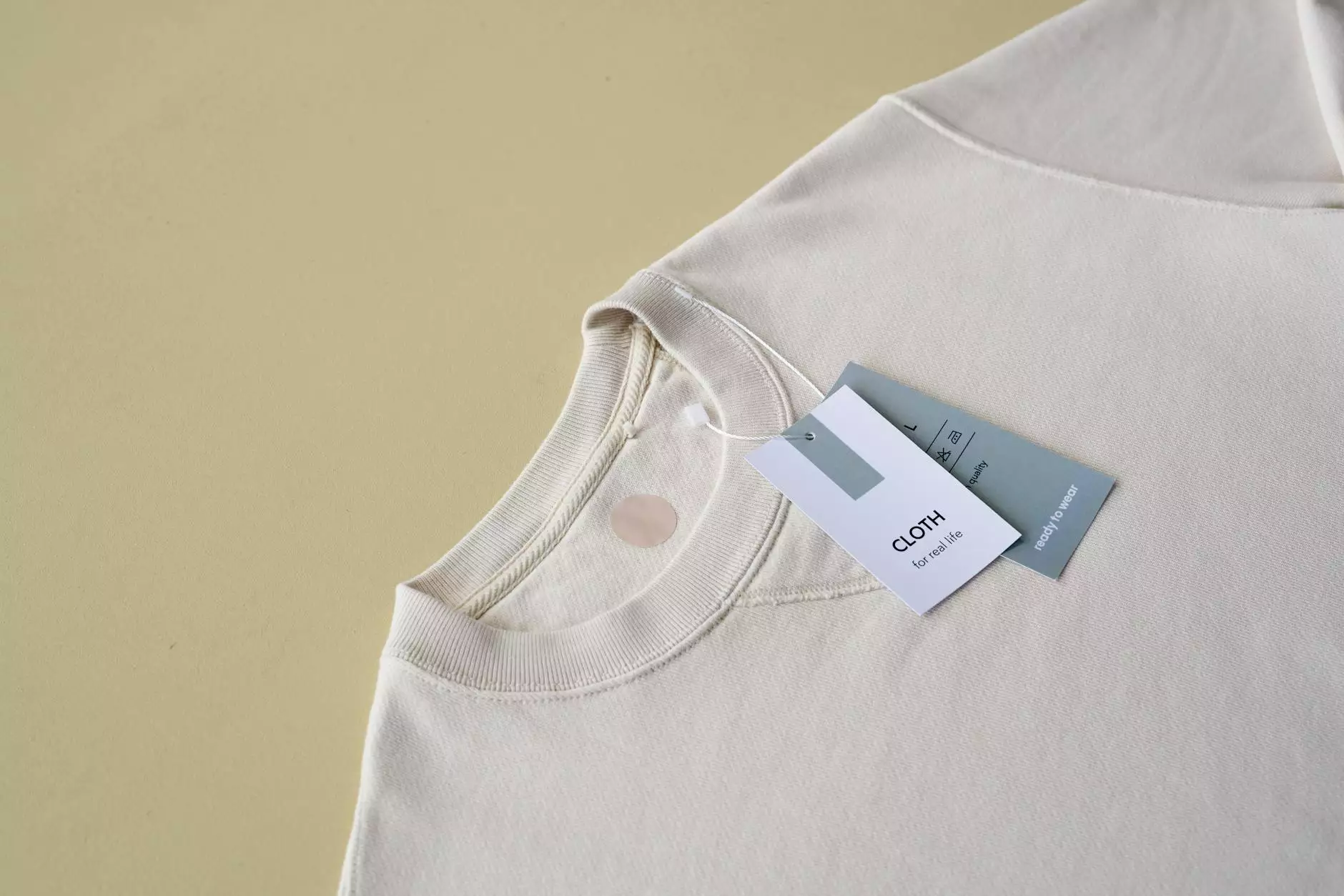
In the rapidly evolving world of technology, machine learning stands as a pillar of innovation and efficiency. Labeling tools for machine learning are essential components that facilitate the data preparation process critical for training algorithms. At KeyLabs AI, we provide robust solutions that lead to superior data annotation experiences. In this article, we will dive deep into the world of labeling tools, their significance, and how they enhance machine learning capabilities.
Understanding the Importance of Data Annotation
Data annotation is the process of labeling or tagging data so that machine learning models can understand and learn from it. The quality and quantity of annotated data directly impact the performance of machine learning models. As such, investing in high-quality labeling tools for machine learning is fundamental for businesses aimed at leveraging AI technologies.
The Role of Labeling Tools
- Efficiency: They streamline the labeling process, reducing the time and effort needed to prepare datasets.
- Accuracy: Tools equipped with advanced features minimize human error, ensuring high-quality annotations.
- Scalability: They allow businesses to scale their data preparation efforts effortlessly, accommodating growing datasets.
Key Features of Effective Labeling Tools
Modern labeling tools encompass a variety of features designed to enhance the data annotation process. Here are some key functionalities that make these tools vital:
1. User-Friendly Interface
A clean, intuitive interface is essential for encouraging user adoption. When labeling tools for machine learning are easy to use, they enhance productivity and reduce the learning curve for new users.
2. Support for Multiple Data Types
From images and videos to text and audio, effective labeling tools must support various data formats. This capability allows organizations to work with diverse datasets and facilitates a comprehensive approach to machine learning.
3. Collaboration Features
Many projects require teamwork. ⚡Tools that enable multiple users to annotate data simultaneously improve workflow efficiency and ensure that all team members can contribute to the quality of the dataset.
4. Quality Control Mechanisms
To ensure the highest standards of data labeling, tools should include quality control features. These can be automated checks or peer reviews that help maintain accuracy and consistency in annotations.
5. Integration Capabilities
Effective labeling tools should seamlessly integrate with existing machine learning platforms and workflows. This integration streamlines processes and optimizes resource use.
How KeyLabs AI Revolutionizes Data Annotation
At KeyLabs AI, we recognize the challenges faced by businesses in the realm of data annotation. Our data annotation tool is tailored to meet the specific needs of various industries, providing value through advanced features and unmatched support.
Comprehensive Annotation Services
Our platform supports an extensive range of annotation types, including:
- Image and Video Annotation: Perfect for computer vision applications such as object detection and image segmentation.
- Text Annotation: Useful for natural language processing tasks, including sentiment analysis and entity recognition.
- Audio Annotation: Enables the development of speech recognition systems and more.
Automated Annotation Solutions
Leveraging machine learning algorithms, our labeling tools can automate portions of the annotation process. This automation not only accelerates data preparation but also allows teams to focus on more strategic tasks.
The Benefits of Using Labeling Tools for Machine Learning
1. Increased Productivity
The efficiency of labeling tools for machine learning significantly boosts productivity. With time-saving features and streamlined workflows, teams can accomplish more in less time.
2. Cost-Effective Solutions
Manual annotation can be costly and time-consuming. By utilizing advanced labeling tools, organizations can reduce costs while enhancing the quality of their data.
3. Enhanced Model Performance
High-quality labeled data translates directly to improved model performance. Our tools help ensure that your machine learning models are trained on the best possible datasets, leading to more accurate predictions and insights.
Choosing the Right Labeling Tool
With a multitude of options available, selecting the best labeling tool for machine learning can seem daunting. Here are some considerations:
1. Specific Needs and Requirements
Identify your organization's unique needs. Do you require image annotation, text labeling, or something else? Understanding your requirements will help narrow down your options.
2. Scalability and Flexibility
Consider whether the tool can scale with your needs. As your organization grows, your data annotation needs may evolve. Choose a tool that can adapt accordingly.
3. Support and Training
A good support system is crucial. Look for tools that offer adequate training and customer support, ensuring that your team can get the most out of the product.
Case Studies: Success Stories with KeyLabs AI
Hundreds of businesses have successfully transformed their data annotation processes with KeyLabs AI. Here are a few noteworthy examples:
Case Study 1: Boosting E-Commerce Sales
One e-commerce platform boosted its product recommendation accuracy by over 30% after utilizing our data annotation platform to enrich their product data with relevant tags and attributes. This led to increased customer engagement and sales.
Case Study 2: Advancements in Healthcare AI
A healthcare institution leveraged our labeling tools to annotate medical images for a diagnostic AI project. This project not only improved diagnostic accuracy but also reduced the time taken for image analysis significantly.
Best Practices for Effective Data Annotation
To maximize the effectiveness of your labeling efforts, consider the following best practices:
1. Define Clear Guidelines
Clear instructions and guidelines for annotators ensure consistency and quality across the dataset. Documenting the annotation process is crucial.
2. Invest in Training
Provide training for all annotators to familiarize them with the tools and processes. This investment pays off through higher quality annotations.
3. Regularly Review and Update Processes
Data annotation techniques evolve, as do machine learning requirements. Regularly updating your processes will keep your annotation efforts relevant and efficient.
The Future of Labeling Tools
The landscape of machine learning is constantly changing. As AI technologies advance, the labeling tools for machine learning will evolve to offer even more sophisticated features. Trends such as enhanced automation, deep learning-powered annotations, and real-time collaborative features are on the rise. Staying informed about these trends is essential for organizations looking to maintain a competitive edge.
Conclusion
In conclusion, investing in labeling tools for machine learning is a strategic move for any business aiming to harness the power of AI. KeyLabs AI offers a comprehensive range of solutions that can transform your data annotation process, leading to enhanced model performance and significant ROI. By leveraging cutting-edge technology and supporting features, you'll be well-equipped to navigate the complexities of machine learning and achieve your business objectives.
Whether you are exploring data annotation tools for the first time or looking to upgrade your current systems, KeyLabs AI is here to guide you every step of the way.